Biodesign Center
Biodesign Center (BDC) is an interdisciplinary research center established at the end of 2019 by integrating multiple computational research groups at TIB. The vision is to empower bioscience with theoretical models and make computational design the core of biotechnology R&D. We develop new algorithms and tools to solve biological problems by adopting novel artificial intelligence methods and computational techniques. Through cross-disciplinary research, we aim to train a new generation of dry biologists and revolutionize the industrial biotechnology R&D paradigm.
BDC develops biological databases, mathematical models, algorithms and software tools for the precision design of enzymes, metabolic pathways and artificial cell factories. BDC manages the TIB high-performance computing cluster (TIBHPC) and the data center for biomanufacturing (BMDC), providing services on computing power, data management and analysis. Based on the established Biodesign platform, BDC collaborated closely with experimental biologists to provide computational support on enzyme, pathway and strain design.
Our Research
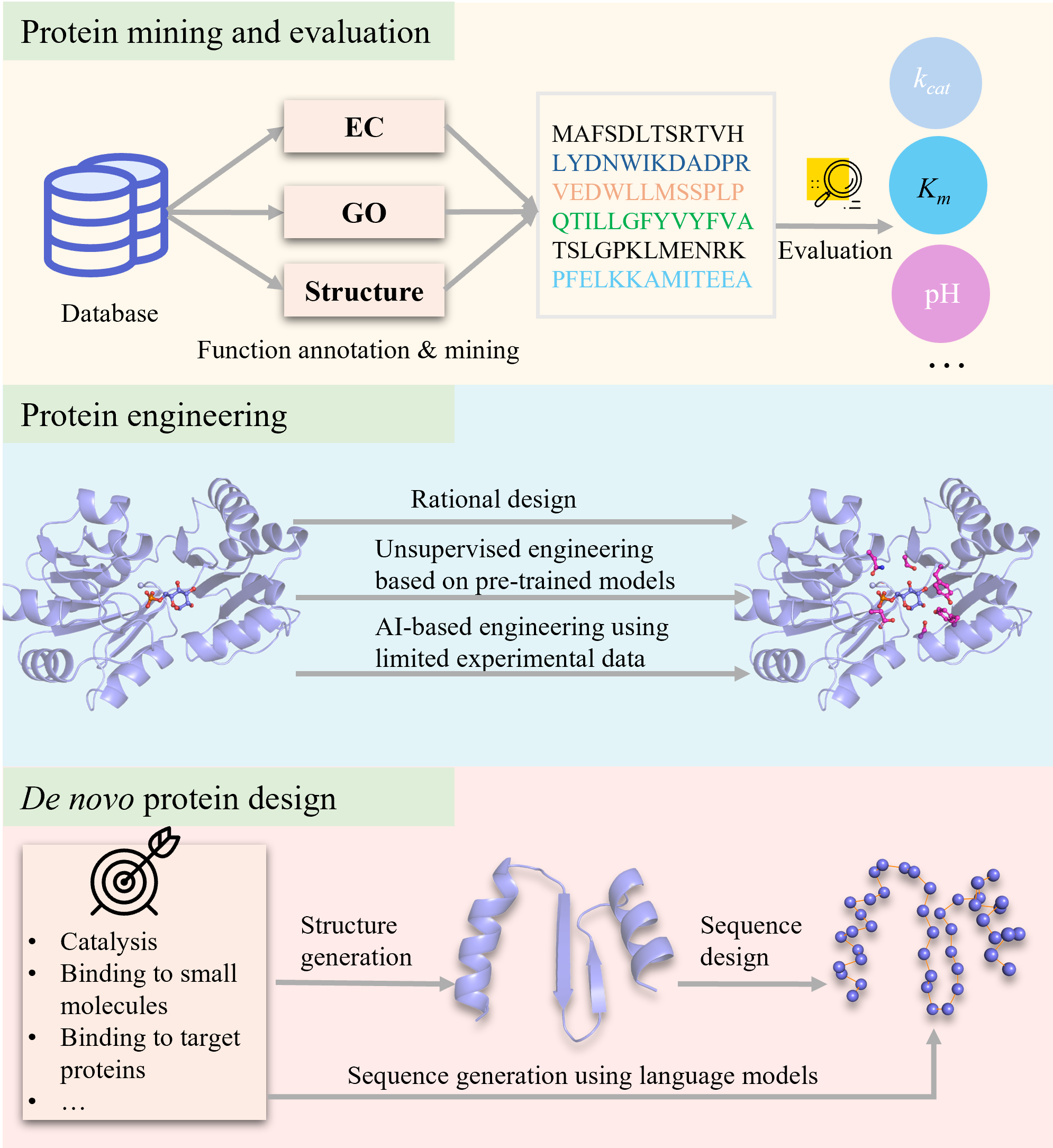
Protein Function Prediction, Mining, and Design via AI and Structural Analysis
Our research focuses on functional proteins such as enzymes and biosensors, aiming to systematically collect and integrate information on protein sequences, structures, functional annotations, reactions, and interacting small molecules to establish core databases. By leveraging artificial intelligence, we conduct large-scale data analysis on protein sequences, structures, and catalytic reactions to uncover intrinsic relationships between protein sequences and functions while developing novel approaches for functional protein discovery to identify proteins with new functions. Furthermore, we construct AI-driven models that integrate multi-source data—including protein sequences, structures, and reaction compounds—to guide protein mutation design. These models undergo iterative optimization through large-scale facility-assisted wet-lab experiments, continuously improving protein functionalities to meet the demands of various applications. Ultimately, we aim to develop an AI-based protein design platform that enables rapid functional protein discovery and design, driving protein engineering toward intelligence and precision.
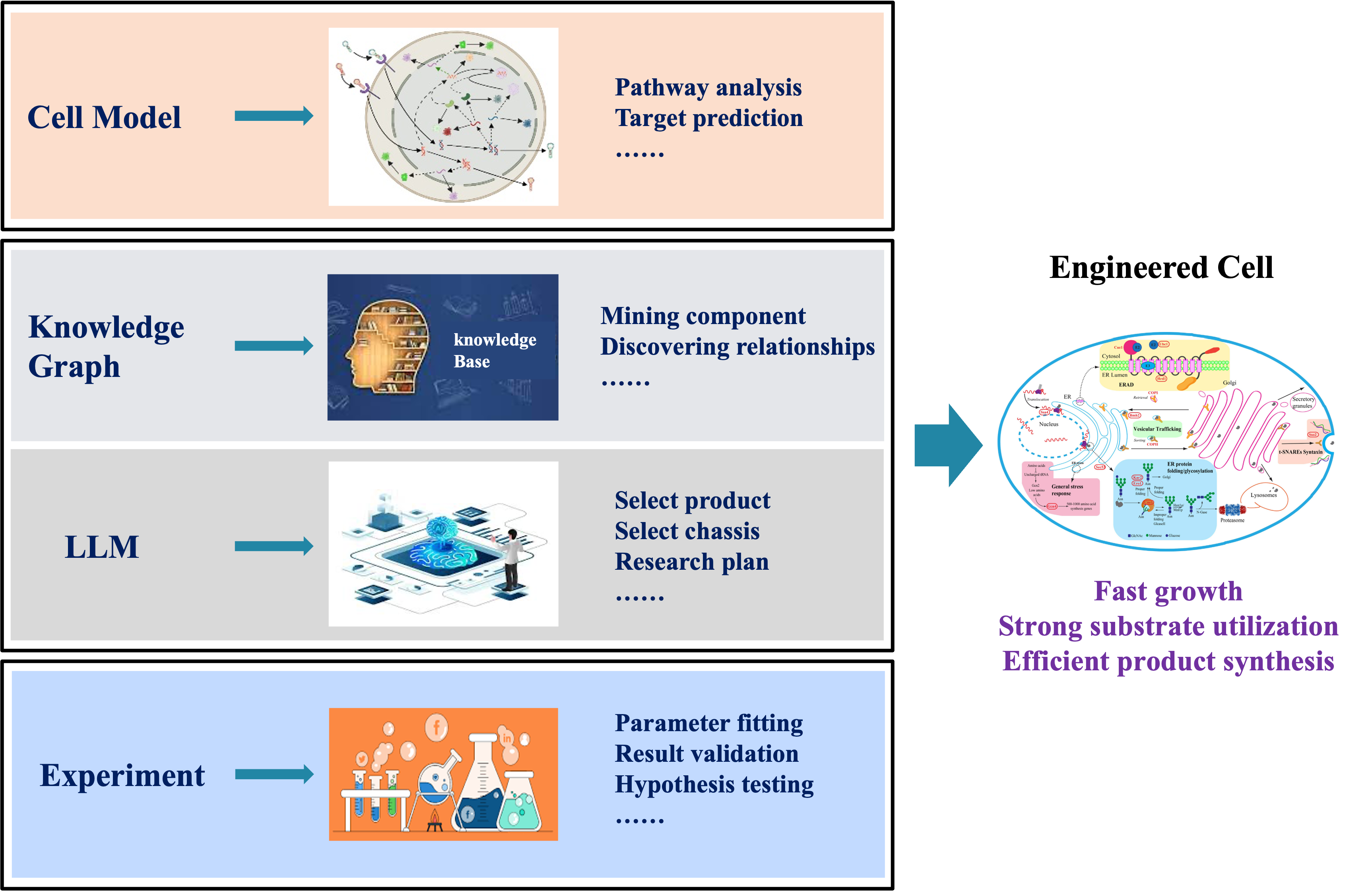
Development of Knowledgebases and Models for Industrial Organisms
Construct a standardized and expandable comprehensive knowledge base for industrial microorganisms by integrating multi-omics data, literature knowledge, and experimental results, providing systematic support for research. Establish an intelligent cell modeling framework by integrating enzyme kinetics, thermodynamic equilibrium, and regulatory network, and other constraint mechanisms with multi-source heterogeneous data of various cellular processes to enhance model prediction accuracy and its application value in metabolic engineering. Develop a “data driven-knowledge interpreted-model predicted” closed-loop optimization system by combining domain knowledge from knowledge graphs with cell model prediction capabilities to build an engineering cell design framework, offering innovative solutions for biomanufacturing.
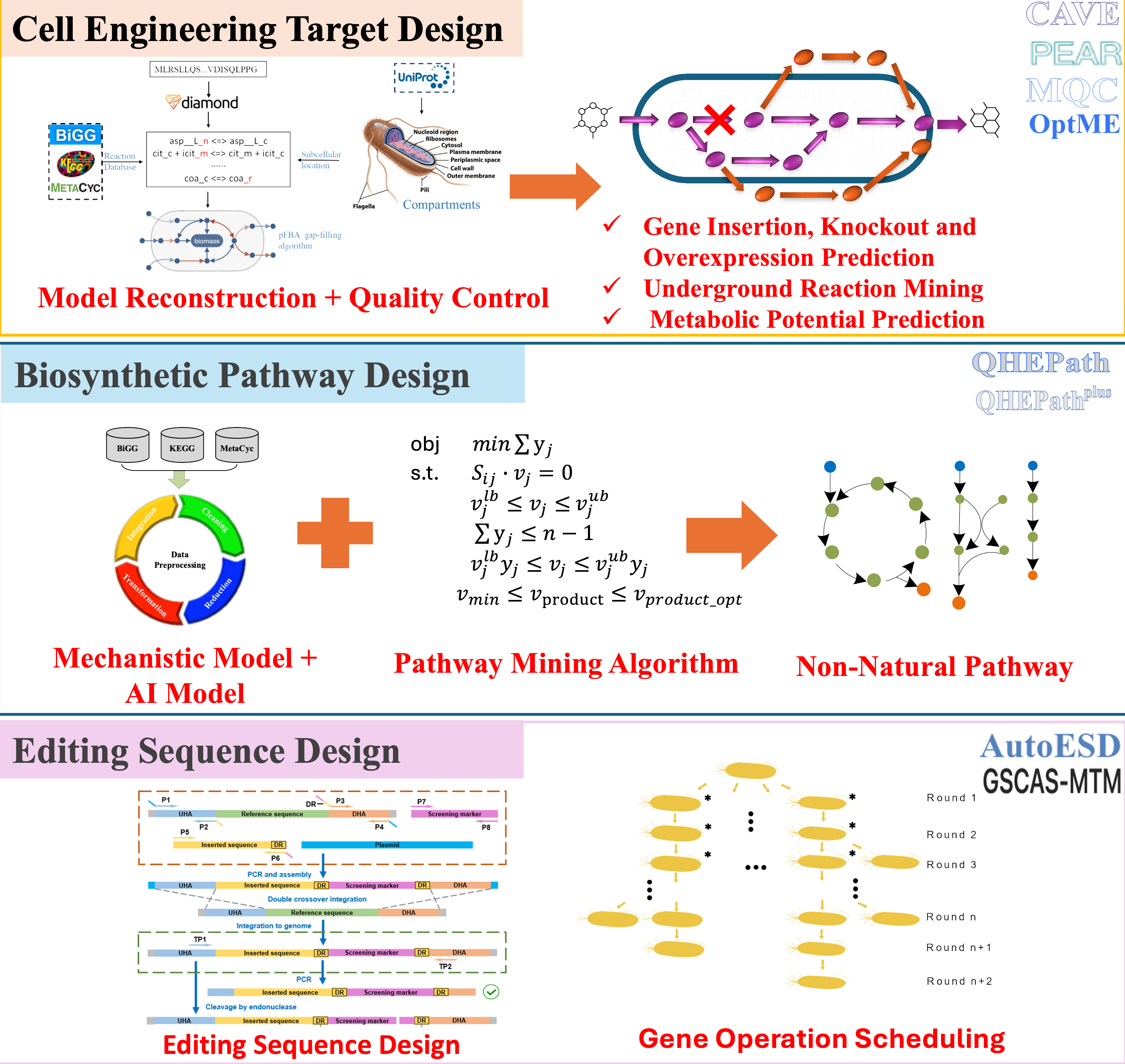
Design of metabolic pathways and metabolic engineering strategies
The team focuses on intelligent biological pathway design, committed to developing a series of innovative algorithms and tools. By integrating metabolic network models with biochemical reaction data, and using multi-objective optimization algorithms, we accurately predict overexpression targets and foreign gene insertion sites, offering diverse metabolic engineering strategies. Combining mechanistic models and artificial intelligence algorithms, we conduct rational design and feasibility evaluation of non-natural metabolic pathways. For carbon fixation pathways and bulk chemicals such as 1,3-propanediol, we predict and assess novel synthetic pathways. Based on the predicted targets and pathways, we develop automated editing sequence design tools and gene operation scheduling methods for large-scale microbial strain modifications, achieving seamless integration in strain design and construction, and facilitating high-throughput automated strain engineering.